What Privacy Practitioners Need To Know About Generative AI
It’s no secret we’re in an AI summer. On every business leader’s mind is how to implement this technology effectively – and how they should consider the legal, reputational, and security risks.
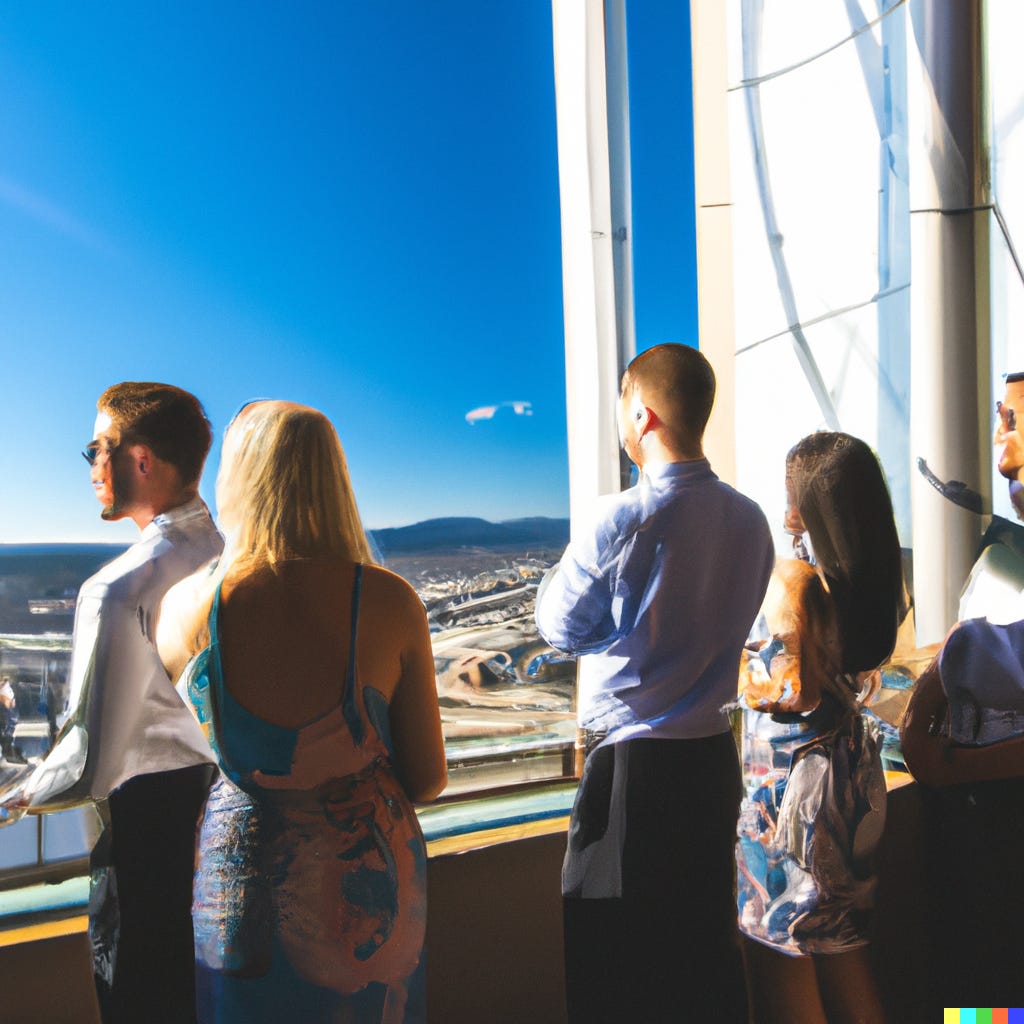
We recently watch Ketch Chief Solutions and Marketing Officer, Jonathan Joseph moderate a panel with Ketch Chief Privacy and Data Security Architect Alysa Hutnik and UC Berkeley lecturer and AI expert, Yacov Salomon on the subject of ethical AI considerations for businesses, which laid out helpful insights surrounding what privacy practitioners need to know about AI.
They also point to the two cornerstone initiatives we’re working on here at the Ethical Tech Project – the technical blueprint (The Privacy Stack) and the nontechnical blueprint (The Commitment to the Ethical Use of Data) – which are particularly relevant as businesses work through the ethical concerns that come with this new era of innovation.
Big picture, there is a big opportunity for businesses: prompt-based interfaces make Generative AI accessible to millions of users who previously lacked the programming skills for traditional AI. However, these models are being trained on public data, and we have not figured out how proper governance of data becomes a part of how these models are developed and trained.
In the meantime, here’s what every privacy practitioner needs to know about Generative AI so that we can chart a responsible path forward and advance toward an ethical internet.
Cross-Functional Considerations
AI is a cross-functional obligation: Data, tech, marketing, legal, and C-Suite teams all need to have input on any decision regarding this technology. There are four key things that privacy practitioners need to know and spread in cross-functional conversations surrounding this technology:
AI is the means, not the end. You still need to decide what you are leveraging the data for and identify the purpose.
Data persists in AI models - The data inputted to train models persists in the model. If you need to take the data out (i.e., if you no longer have permission to use it), then you must have the technical infrastructure that enables that.
Third-party AI captures data, too - Models also carry obligations and models do not negate the need for governance.
Ethical Data Means Ethical AI - The outputs of the models have effects: discrimination, biases and other negative outcomes that need to be managed and neutralized. Privacy extends into ethics, and they share a consumer protection regulator (The Federal Trade Commission).
Legal Considerations
Most of the time, the bulk of the issues have often fallen on privacy counsel because personal data is at the heart of AI. In these cases, the most critical thing to consider is identifying all the potential risks you can foresee and executing a plan for how you manage those risks. There are three key things to know to execute this in practice:
Understanding what the technology is specifically being used for, so you can issue-spot appropriately. The reason for implementation can’t just be to use AI for AI’s sake and there needs to be transparency and fairness in outcomes.
AI initiatives are dead-on-arrival when access to data, especially personal data, is limited because of privacy and compliance gaps. Depending on how sensitive the data is, businesses need to give a consumer the right to withdraw or delete when there’s a foreseeable negative consequence.
Implement controls for all contracts. Compliance today is focused on contracts – i.e. legal agreements with vendors and partners that they will treat the personal data they receive responsibly. But controls related to these contracts are equally important, which are the technical infrastructure that helps enforce customer’s privacy choices across your product’s ecosystem.
Ethical Use of Data Considerations
We’re seeing data become a lot less about individual interactions and more about how it gets multiplied inside intelligent systems. Because of this, the majority of data in the public sphere will live inside models – and we must progress from there in terms of privacy.
At the Ethical Tech Project, we’re focused on what businesses need to consider about data before we throw it into a model, and especially, what the outcome is.
Our work at ETP started with addressing a gap in the market: How do you actually design technical systems to be both compliant and future-proof for everything that’s coming? So we first designed:
The technical blueprint. We went around the industry and spoke to stakeholders and legal minds to put together a privacy architecture, ThePrivacyStack, which is an open-source project you can find on GitHub today.
But, the feedback we heard from organizations what super positive, and they’d like to implement it, but not for years because it's not a business priority. So, we understood that we needed to bring the entire organization on the journey, which is why we developed:
The non-technical blueprint. These are guidelines for Board and C-Suite level executives to consider as they embark on the journey toward responsible data use. It outlines five principles: privacy, agency, transparency, fairness and accountability that leaders must adopt to advance toward data stewardship.
We’ll be digging into each of these blueprints in future posts – stay tuned!
What We’re Reading
Tecnology just enables whar we do. If we don't like what technology enables us to do, we should question what we are doing. In a word: eudaimonia.